While things are normalizing a bit in the automation space (specifically marketing automation), things are really heating up in the Artificial Intelligence space for marketers. From copy creation to full-on video production, AI now touches every aspect of marketing in various ways.
It's clear that AI has changed the present and will continue to shape the future.
However, what's not clear is the relationship between automation and artificial intelligence. A lot of this confusion stems from the constant evolution of the AI space, but frankly, the rest is due to ignorance. Many simply don't understand how the two work separately before trying to grasp their collective function.
Hopefully, this blog post will shed some light.
Disclaimer: For all the heavy AI folks reading this, I am in no way an AI expert. I'm an experienced digital marketer (and Automation Service Provider) specializing in using cutting-edge tech to increase efficiency and profitability. So, I might misuse terms or not speak 100% accurately about AI, but for the general population, the difference is undetectable.
Let’s start with some basic definitions of both, fitting the context in which I'll be explaining them: “thinker” and “doer.”
Artificial Intelligence is the process of automating thinking (and learning). The more accurately it can emulate human cognition at scale, the more useful it becomes. AI can process more data and faster than a human brain ever could.
Although automation is used in the process of training AI models, that's not the function I'll refer to here.
Instead, think of automation as the automatic movement of data, usually from one app to another. Unlike AI, automation is very “dumb.” It doesn't do any thinking for you; it relies on your instructions for what to execute continuously.
So, if AI is the brain, then automation is the hands. AI is the thinker, and automation is the doer.
Taking it a step further, AI requires an input—a prompt—as the starting point of its thinking (or generating). This is usually done manually but can also be handled by automation.
Referring to the image below, you see that automation is represented by two arms. One provides the prompt to AI, and the other places the result from the prompt into the desired software.
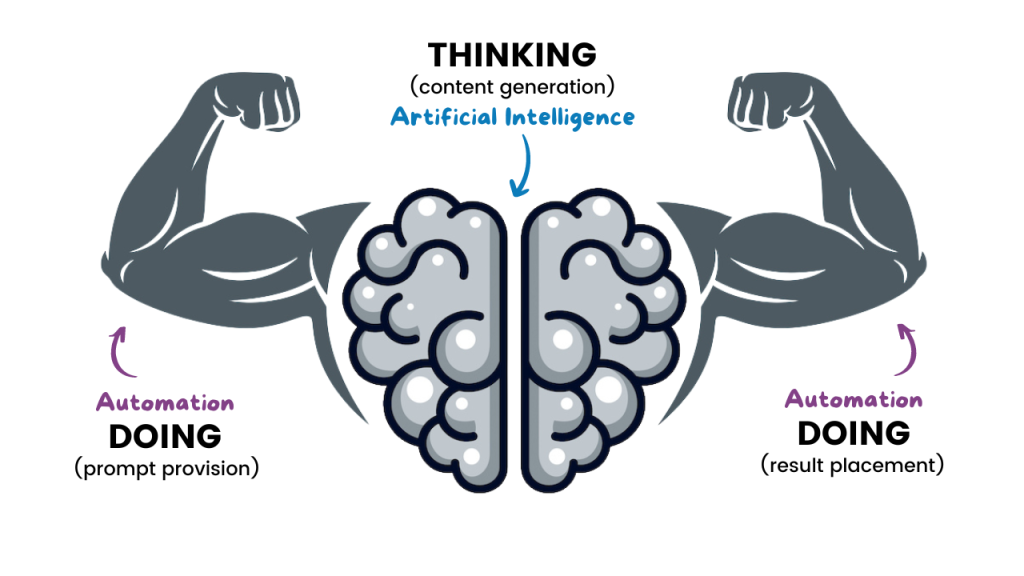
This is the relationship between the two! Let’s look at a couple of examples:
Example #1 – Auto Prompting
Below is a screenshot of a Make scenario I created to automate a step in my podcast post-production process. Once an episode is marked as “Ready,” it triggers this workflow to:
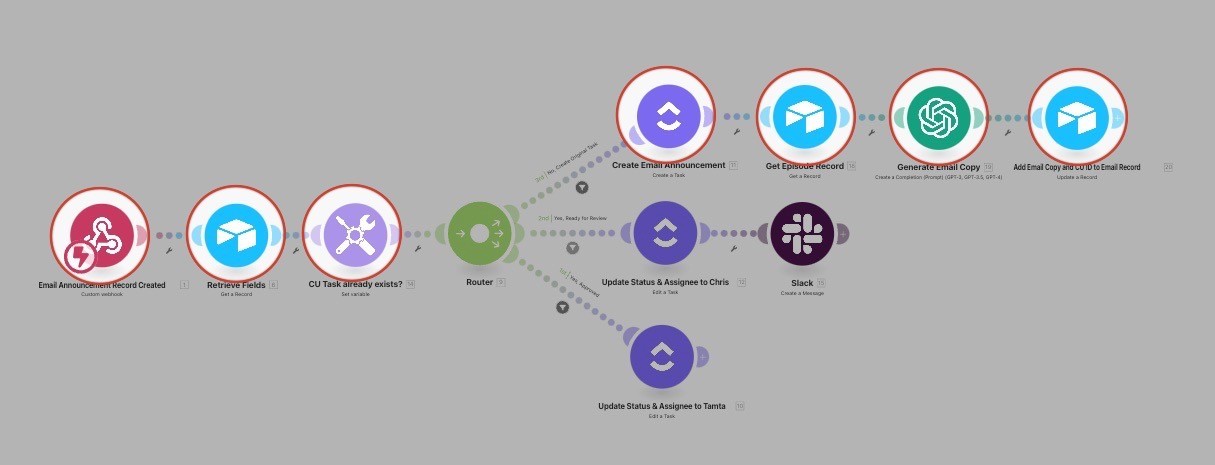
- Get the episode content from the Airtable record
- Send that data, wrapped in a prompt, to ChatGPT
- Place the results into another field inside Airtable
Here, instead of logging into my ChatGPT account and copying and pasting the content, automation handles that step.
Moreover, I’m paying much less to generate a response by using automation and leveraging the API than I would by paying monthly for ChatGPT.
A mastermind friend recently showed me a similar process where he used Airtable to Leonardo (recently acquired by Canva) via the API to automatically generate video content.
This is where I see a huge opportunity for evolution in the AI space. Currently, not many platforms directly integrate with tools that can produce prompts (like Airtable or a Spreadsheet). Hence, the need for tools like Make.com (or Zapier) to pass the data. More native integrations with AI tools mean easier production and higher efficiency of outputs.
Currently, companies are tackling this challenge by adding AI to their platforms. It’s now rare to sign up for software that doesn’t have some native AI capabilities (usually requiring extra payment).
Whether through direct integration with external tools or built-in AI capabilities, the goal is to easily generate meaningful AI outputs with minimal prompting.
Example #2 – Auto Bots
Another way to illustrate the relationship between automation and AI is through agents (or bots). An AI Agent is a self-sufficient robot designed to execute a sequence of tasks (which can involve cognitive processes like driving, flying, checking out, etc.).
When these capabilities are deployed by software on your computer, you get what's called Robotic Process Automation (RPA). This type of automation can log into accounts for you, copy and paste data, and even analyze and correct data before pasting.
Though still a new technology (and pretty pricey), we can expect lower costs and higher accessibility over time. Here are some use cases where companies are currently using RPA:
- Data migration/entry – transferring paper forms to digital
- Customer Support – consolidating all information about a customer on a single screen for the rep
- Sales Team Support – handling administrative tasks such as updating the CRM system and inputting data into sales dashboards
In closing, the automation landscape is changing. While “garbage in, garbage out” still holds true for your approach to automation, you're no longer limited to your own intelligence to get the desired results. This isn’t permission to be reckless but rather a mandate to be more responsible.